Summary
In this working paper, we develop the following thesis. In the not so distant future (a decade or two), medicine will be largely governed by algorithms — highly deterministic clinical pathways characterized by a high level of reproducibility of care — that will be developed and improved by providers. These algorithms will include individual patient preference branch-points but not individual provider preference. As a result, payers and providers will agree on coverage on the basis of a set of algorithms and a process of how they should evolve; providers will be paid on a fee-for-service basis for following the algorithms; and finally, what is not algorithmic will be managed as explicitly experimental. We show how this evolution fits with long term trends, and potentially resolves a number of long-standing tensions in healthcare, in particular, the principal-agent problems that beset the patient-provider-payer triangle.
Core agency issues in the provision of healthcare
The reason the Hippocratic Oath exists stems from an early recognition of agency issues in the practice of medicine compared to other professions. The vulnerability of a patient, the asymmetry of information, the economic incentives, the discretion in the hands of the physician, and the potential urgency of acting, all combine to invest providers with immense leverage. Various approaches have been used to mitigate this imbalance of power: intensive ethical indoctrination in medical schools, professional societies’ codes of conduct and policing, payer organizations’ oversight, all with a limited degree of success.
To crystallize the problem, consider two ends of a spectrum of possible payment mechanisms to providers. In fee-for-service (FFS) a provider has a financial incentive to over-treat whereas in a capitation system, a provider has a financial incentive to under-treat. Honorable small practitioners often do the right thing, but some demonstrably don’t and, when a large organization is at work, it becomes a much trickier proposition for individual staff to act independently of the interest of their employer. For instance, there are widely different behaviors when it comes to ordering imaging studies in the emergency department[1]: a patient with rich private insurance benefits will get liberal MRIs (under FFS), an indigent case will not (this is essentially capitation under the charity care funds).
Another helpful perspective on this conundrum is that of management theory. Building on the foundational work of Peter Drucker, there are essentially two basic methods of management:
- Management by objectives: the manager assigns high level goals, but leaves wide latitude on the activities to reach those goals
- Management by rule-based activities: the manager assigns a specific schedule of activities that need to be accomplished (sometime depending on additional inputs)
Each of these management approaches have a natural association with different incentive schemes:
- Management by objectives: reward based on outcomes
- Management by rule-based activities: reward based on work accomplished
Obviously, matching a management approach with the wrong incentive scheme is going to be sub-optimal. Thus rewarding on outcomes someone who is managed by rule-based activities is unfair since they might properly accomplish their assigned set of activities but not achieve the goal, or vice-versa. Similarly, rewarding on work accomplished by someone who is managed by objectives will not give the desired result. It is to the latter that the traditional FFS compensation mechanism corresponds; health practitioners are given the broad objective of “making the patient better” and then paid on the basis of individual activities.
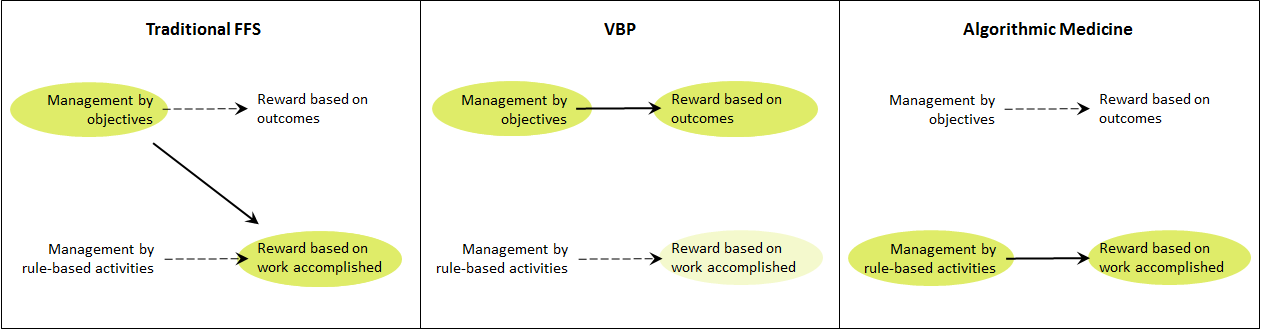
This management approach/reward mechanism mismatch has been widely identified in health policy circles, and current prescriptions to rectify the situation, including with the ACA, are to shift the reward system to one based on outcomes based (Exhibit 1). But as the ACO experience has shown so far, this is not easy to do — in terms of outcome metric definition and settings, in terms of risk-adjustment, and in term of defining a population in scope when care is obtained across health system boundaries.
There is an alternative path to addressing the mismatch in management approach/reward mechanism; it consists in changing the management approach rather than the reward mechanism[2]. In that line, we envision the practice of medicine evolving to one that follows prospectively defined algorithms of care. The great benefit of such a scheme is that it allows the use of FFS because of greatly decreased agency issues — and therefore it does away with the complex issues of risk-adjustment and patient allocation that bedevil outcome based payments. As we argue further in the paper, it also enables a very different approach to quality management. It does create a new set of challenges mostly around creating, implementing, and evolving algorithms but we argue that resolution of those issues is naturally aligned with current trends in healthcare and technology.
Trends driving toward algorithmic medicine
Before going further let us be more specific on what we mean by algorithmic medicine. In this framework, an algorithm is a prospectively well-defined pathway that is followed by health practitioners as they diagnose and treat their patients. Note though that sometimes, an algorithm may have decision points which depend on patient preference: for instance in prostate cancer, the alternatives of radiation therapy vs. surgery vs. watchful waiting. However, the algorithms would eliminate “provider preference[3]” as a dimension of choice, and in that sense the main feature of an algorithm is reproducibility — the same patient seeing different physicians following the same algorithms will be cared for in the same way.
Algorithmic medicine fits into a broad arc of the evolution of medicine (Exhibit 2). Like many industries, we are now at the verge of a new era where many activities that used to be based on human management are now ready for transition to IT-supported automation. Key to keep in mind is that in healthcare as elsewhere, this is not only about an increase in productivity, but also about a gain in quality by decreasing uncontrolled variability.
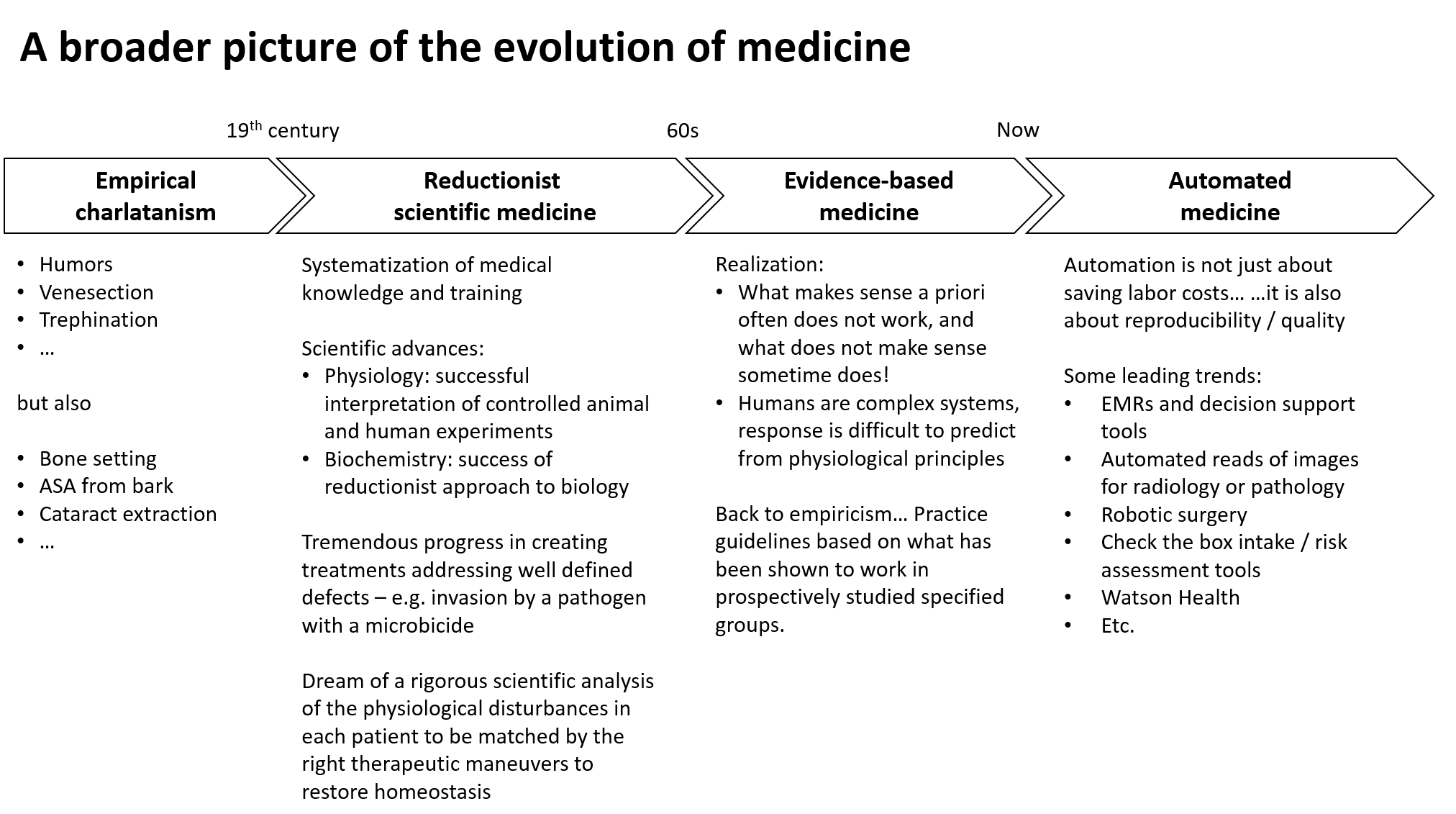
At a more micro level, several trends are converging to push medicine toward “deterministic” care.
The first trend is task shifting, in particular to mid-level practitioners (NP, PAs, midwives) especially in rural areas which fail to attract sufficient MDs and in retail clinics where the expected price point is low. For quality assurance and also concerns of legal liability, these practitioners often use a highly scripted approach to patient care, which also include clear boundaries of care beyond which referral to a higher level of expertise is mandatory. As the role of non MDs continues to increase in the primary care arena, there is an expectation that the library of algorithms covering their activities will continue to expand.
Another powerful trend is the increasing fragmentation of care driven by specialization, and a transition for many physicians from a way to practice that was a “calling” to a functional role. This has two important consequences. First transitions of care are more frequent and often take place between professionals who have a limited relationship — this makes the development of standards necessary so that balls don’t get dropped due to false assumptions about who is doing what and when (a common source of errors in medicine). Second, the acumen of physicians is decreasing because less and less they have a full longitudinal experience with the patients they manage. Among other issues, they cannot learn from their mistakes, because they don’t see their mistakes play out. This has always been the case for the Emergency Department, where health professionals see patients once for a few hours and where algorithms have been a vital element of improvement — but it will eventually be the same for most areas of medicine.
Yet another trend is driven by the sheer complexity of data to be integrated at the level of a patient: for instance, a single patient may now be on ten or more different medications — and checking for interactions is something that is done by a computer algorithm, not by the practitioner thinking about it. Similarly, HIV sequencing has become a determining factor for anti-retroviral therapy whereby mutation X indicates that treatment A is warranted unless mutation Y is also present in which case it should be treatment B etc. etc. With human genomics slowly getting integrated in care, this complexity is only going to increase, and machine-supported algorithms will be the only way to manage[4].
This is not to suggest that this shift will happen all at once. Clearly, there are some areas in medicine where algorithms are easier to apply (e.g. consensus around an evidence-based algorithm already exists within a relatively narrow band of variation, and the system supports following the algorithm) while there will remain areas where the standard of care is far from near. The point though is that as one thinks about the total quantity of care provided to a population, over time, a greater share will be (or should be) algorithmic (see Exhibit 3)[5].
Constructing, improving, and implementing algorithms and the relationship with experimental medicine
Because of their expertise and also their commitment to patients, providers need to take the lead in developing algorithms (with input from other stakeholders). In fact, we believe that in the future, algorithm design and improvement will be the highest value add activity within the entire health sector.
What we envision is that providers will adopt a certain set of algorithms chosen from a portfolio of well-respected standards developed by top clinical institutions. Those would then be customized for the local practice environment. Payers would contract with providers on the basis of:
- An agreed set of prospectively defined algorithms
- An agreement on the process through which the algorithms will evolve and improve
- A charge master that will link to algorithms to establish a FFS schedule
- An audit methodology to make sure the algorithms are properly implemented (together with a definition of what is an acceptable rate of deviations)
- Quality factors to be included in the compensation scheme
- A process to manage situations for which there are no accepted algorithms
To be clear, in this context, “Payers” is meant to be the entity bearing financial risk or its representative (so that sometimes, “Payers” are health systems themselves rather than insurers, with the providers being one level down — i.e. hospitals, physicians, etc.).
What should happen in a situation not properly covered by an algorithm (or without an algorithm)?
In such a scenario there are two possibilities:
- Either the practitioner believes the course of management is clear and then the algorithm needs to be updated so that it includes that insight rather than bypassing the faulty algorithm[6],
- Or there is truly no clarity on what to do, and then subsequent course of action has to be managed as an experimental pathway. This means that it is documented and structured in a way that it can lead to actual learning.
What actually happens now outside well-structured care pathways (still the largest part of medicine)?
- Enormous variation in care between practitioners has been abundantly documented over recent years, be they regional, or dependent on practice settings[7]. The same patient may go to three practitioners with, say back pain, and get three completely different courses of treatment — but those three practitioners will nevertheless all think that they are within standard of care.
- Or there is no clarity at all on what to do, in which case practitioners try things – but outcomes are never documented in a systematic way so there is no good way to leverage the experimentation as a mechanism to advance knowledge — an enormous waste of potentially useful information.
The reason the current system muddles through is that it is to some extent self-correcting – as long as patients keep getting worse, they keep coming back until the right intervention takes place[8].
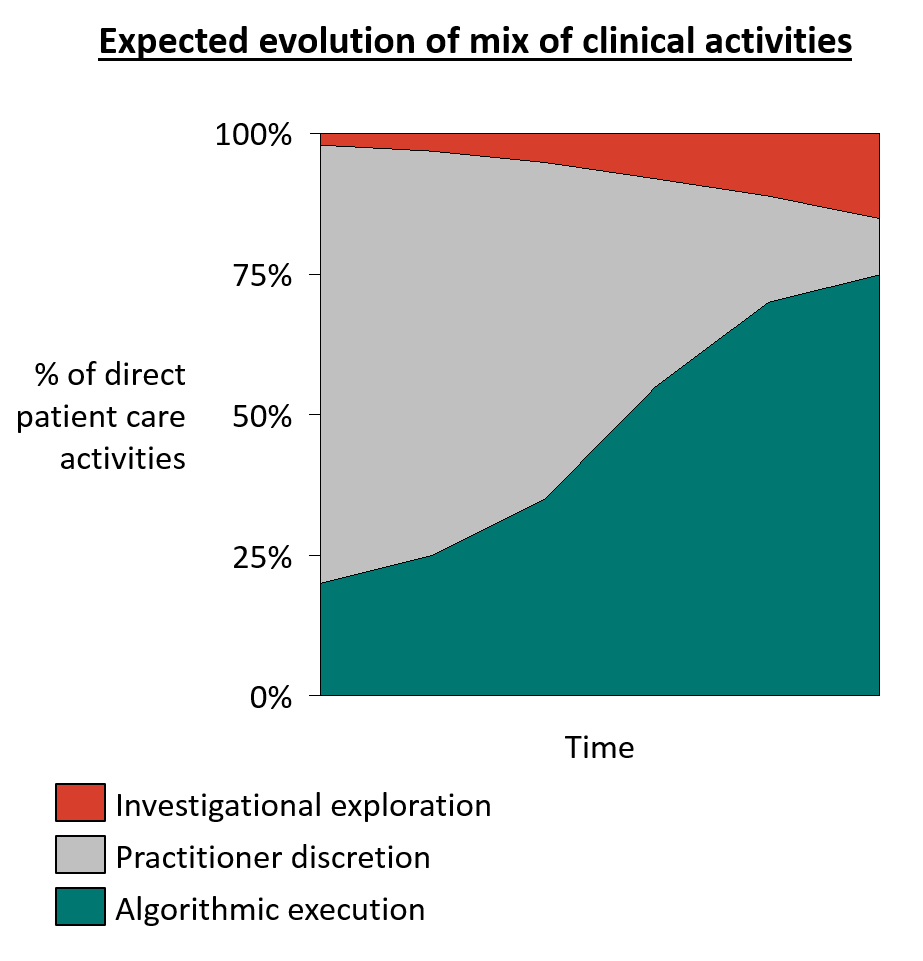
The evolution that we predict is shown in Exhibit 3. Under the current state, a large fraction of patient care activities are performed at the practitioner’s discretion with major variability. Over time this discretionary domain share will shrink as more and more pathways of care are codified into algorithms that are then executed and improved by practitioners. In the many areas where there is true ambiguity currently resolved through practitioner preference, there will be a gradual shift to formally designate and manage activities as experimental so that they can be a source of learning for the field at large. There is potential for academic medical centers (AMCs) to become the leading drivers of this process.
Challenges for algorithmic medicine
The biggest challenge in the transition to algorithmic medicine is the change it implies in the nature of the role of physicians, and in particular in the self-perception of the profession. There is no denying that the last 30 years have been wrenching ones for physicians and any further change felt to yet increase a pervasive sense of lack of control is not going to be well received. The degree of stress in the profession is particularly strong in areas of medicine where there is a strong sense of accountability for the ultimate fate of the patient (e.g. in primary care) coupled with a sense of being hogtied by a complex and often unpredictable bureaucratic system.
For this reason, it may be informative to look at another group of proud professionals that has made this transition: pilots. In the beginning, airplanes were made of cloth and wood, pilots flew by the seat of their pants, and disaster was common. Gradually the profession disciplined and systematized itself, and today any flight falls under one of two well-regulated categories:
- VFR (Visual Flight Rules): essentially allowing the pilot to do whatever they want except for what is forbidden
- IFR (Instrument Flight Rules): where key actions by the pilot (e.g. heading, speed, altitude change) are determined by preset procedures, or traffic control instruction or clearance
Nowadays, almost all commercial flights are conducted under IFR, and the argument of this paper is essentially that physicians (or those who will do the work that physicians do today) will need to fly IFR too. Over this transition, commercial pilots have morphed from bold individuals to trusted professionals who operate by the book with high reliability in a complex environment. The peace of mind of a commercial pilot stems from a sense of a smooth adherence to the procedures of flight and a confidence in their training to manage exceptions and emergencies.
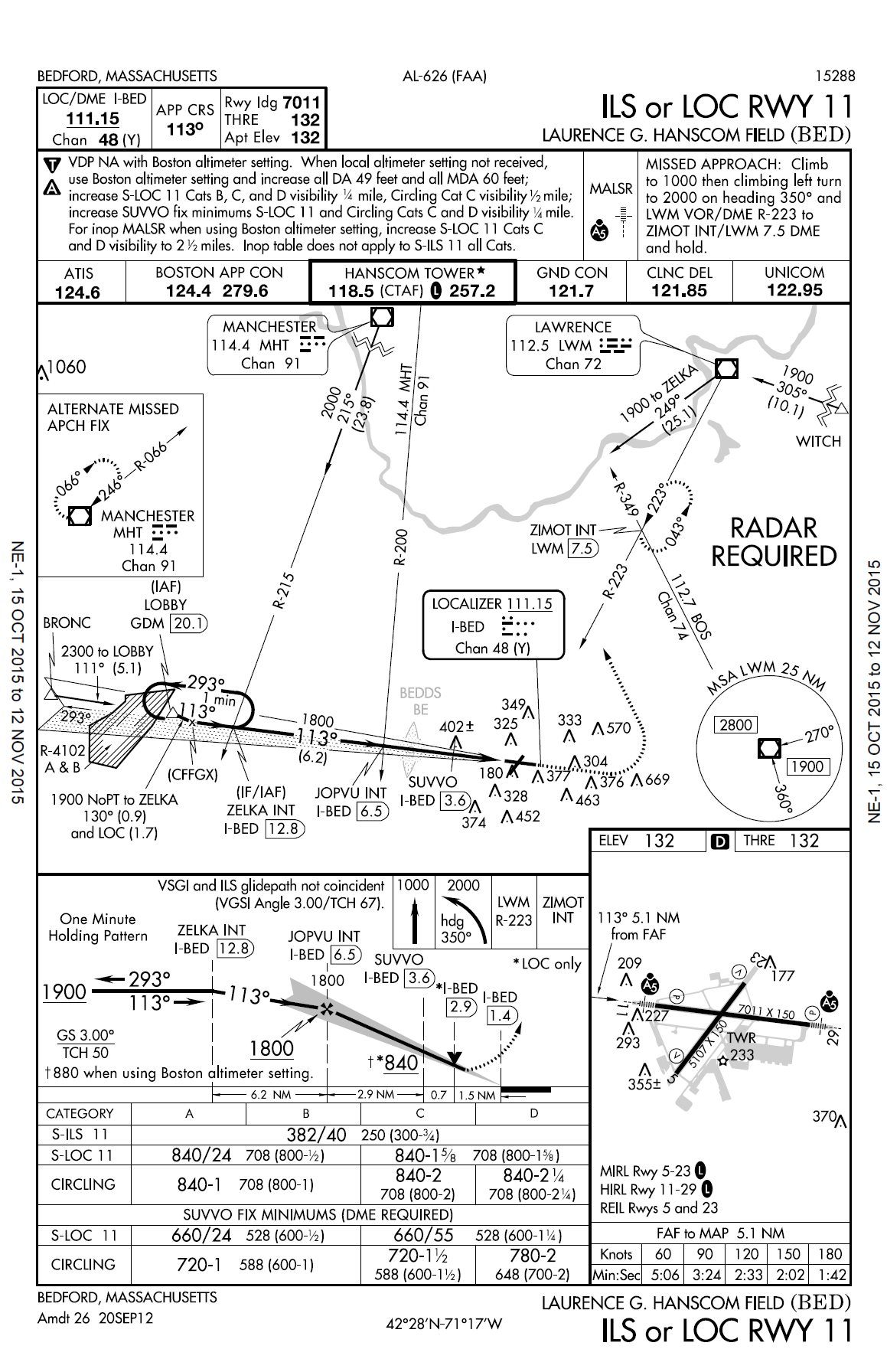
As was recognized by Toyota long ago, a key motivator for staff is to ensure their engagement in the approach to their work. And this is where perhaps the transition in expectations about the practice of medicine ought to lie: instead of creative approaches to patient care, creative input to design and redesign care pathways. Textbooks of medicine are full of diseases named after physicians who noticed a pattern among their patients. While these days are past, the new frontier of opportunity for those who want to have an impact on the practice of medicine will be to create improvements in the care process. Another challenge to algorithmic medicine is one of feasibility: medical conditions and their contexts are so diverse that one might think it impossible to create a reasonably comprehensive set of algorithms. Once again the example of aviation may be useful — Exhibit 4 shows the instrument approach plate for one runway at a small airport located near Boston. It offers the pilot a full set of instructions including contingencies (missed approach). There are thousands of those plates; they were created before the age of IT, and they are reviewed and revised on a regular basis. Management of patients is of course more complex, but not by many orders of magnitude. A lot of the heavy lifting has been done through the creation of ICD and CPT codes. The availability of EMRs with basic big data analytic techniques should allow the creation of first draft of algorithms. It would then be up to experts to review, and practitioners on the ground to input refinement to get to a product usable at scale.
It is however the case that some elements of care connected to the approach to behavioral change will be less amenable to algorithmic solutions. For instance, it is well and good to put an intervention such as smoking cessation counseling into an algorithm of care, and even describe some steps of that process, but the effectiveness of that counseling is highly dependent on an adaptive approach to patients which is beyond the reach of algorithms (at least until natural language-capable AI appears). There is a reason salesforces are paid on outcomes rather than to follow a script.
Conclusion: algorithmic medicine in context
Although typically mentioned using different nomenclature, the trend toward the algorithmization of medical practice has been described by many, such as Atul Gawande with emphasis on checklists (another tie in with aviation procedures) and a comparison with the operating model of the Cheesecake Factory[9]. Some have actually begun to implement this process under the SCAMP framework[10] Similarly, Clay Christensen, Eric Topol, and others have also touched on this evolution in their writing. However the work of all these authors has focused on the impact of technology, as well as the opportunity to improve quality and efficiency through standardization wherever possible in a way that leverages IT solutions and the learnings from management approaches in other industries. We are in general agreement with these lines of argument, but the perspective of this paper adds emphasis to two other points that we consider critical.
First the algorithmic approach offers for the first time, opportunity to meaningfully diminish the outsized agency issues that bedevil healthcare in a market environment. It certainly does not eliminate those issues entirely, but we would argue that it makes them comparable in magnitude to what is found in other sectors. In many ways, these agency issues are at the core of what makes health care policy so difficult to grapple with, and with some degree of resolution, there is a potential to make much faster progress as the root cause of mistrust between the various actors is mitigated.
Second, this work makes the claim that most of the clinical situations for which the state of knowledge is insufficient to enable coverage by an algorithm deserve to be dealt with under experimental standards of documentation. When a practitioner tries something, the information that is generated from this experiment is valuable, and should be usable for the creation and/or betterment of algorithms of care. We are well aware that this approach would require significant adjustments in the regulatory framework — it is not possible nor desirable to bring in an IRB to approve every experiment that would result from such a stance, and some sort of safe-harbor criteria would have to be designed.
Finally, there is a significant question in how to properly incent and compensate the work of creating algorithms of care, which as we have said previously, we believe will be by far the highest value adding activity in the health sector. This is where we believe a value-based framework would come into play optimally (rather than at the level of actual provision of care where we have made the argument that FFS for following care algorithms is optimal). In this context, the question is subsumed in the topic of funding of clinical research taken in its broadest sense, i.e. “how to improve patient care”, be it by developing new insights through prospective experimental studies, or through mining existing datasets, potentially using machine-learning and big data approaches. In the current state, the lion share is funded through two mechanisms: public funding and development activities by commercial pharmaceutical and medical technology companies. Interestingly, for the commercial side, while the major effort (and value) is usually the development of information that support use of products, the monetization of this effort depends not on the intellectual property acquired during the development process, but rather on patents for the product themselves. In order to speed up and strengthen the drive to structure improved algorithms of care, it will be necessary to find ways to better reward the intrinsic value generated by such work. That is a hard problem which we will try to address in further work.
[1] Anecdotal evidence is common among physicians (ordering the “panscan”), but also documented in the peer-reviewed literature, e.g. see Brinjikji et al., Disparities in Imaging Utilization for Acute Ischemic Stroke Based on Patient Insurance Status, AJR, 203, pp 372-6, 2014
[2] Such an attempt was actually made in a crude way during the managed care failed experiments of the late 80s and early 90s with payer-based case managers muscling into individual care decisions — especially egregious was the fact that this was often applied in a context of capitation leading to acute issues of agency.
[3] With the side benefit of mitigating unconscious/undeclared bias which has been shown by many to affect disproportionately certain disadvantaged populations of patients.
[4] A recent review in the NEJM (Dohner et al. 373:1136-52, 2015) on leukemia (AML) shows 13 major types of mutations (hence 2^13=8196 possible genetic signatures) and approximately 50 drugs in development most of which are targeted to specific molecular defects.
[5] A noteworthy variation to this process exists in some low-income countries where health sectors are being built from the ground-up on an algorithmic foundation. Given few physicians and nurses are available, community health workers with limited formal education are trained to provide care by following well-defined protocols which are regularly reviewed at the national level based on data from the field, evidence from new research, and finally availability of resources (drugs etc.). This has worked remarkably well in Rwanda where life expectancy at birth has risen above age 60 despite a mean GDP per capita of less $2 per day.
[6] The process by which processes are systematically improved rather than bypassed when leading to defects is called Total Quality Management in the manufacturing sector, and in terms of economic impact, has been one of the leading management innovation of the 20th century
[7] e.g. see the work of the Dartmouth Atlas of Healthcare highlighting geographic variation care, or for an interesting study of how provider self-interest influences care in prostate cancer see Mitchell, Urologists’ Use of Intensity-Modulated Radiation Therapy for Prostate Cancer, NEJM, 369:1629-37, 2013
[8] A notable exception is mental health where the likelihood of a patient returning for care may not increase with the level of illness
[9] Atul Gawande in The New Yorker Dec 10 2007 (Checklist) and Aug 13 2012 (Cheesecake Factory)
[10] E.g. see Sox and Stewart, Algorithms, Clinical Practice Guidelines, and Standardized Clinical Assessment and Management Plans: Evidence-Based Patient Management Standards in Evolution, Acad. Med., 90:129-32, 2015; and also www.scamps.org