Working Paper
Summary
Drug companies are naturally incentivized to price their drugs under assumptions of optimal clinical value, i.e. as high as possible. Payers react to this by setting stringent conditions for patient eligibility for coverage of those therapies. As a consequence, patients who do not meet these conditions do not receive those drugs even though they could derive benefit, albeit not of a magnitude that would justify the cost. Here we lay out a population health based scheme by which payers and drug companies can design a system that ensures access to a drug to a larger group who could benefit at a cost that is adjusted for the aggregate benefit to the group. While there is some relation to the general family of rebate and/or discount agreements commonly entered between drug manufacturers and payers, this scheme is different in that it adjusts price by taking into account the patient mix and related incremental health benefit. We sketch out this approach using an illustrative toy-model in the setting cardiovascular drugs such as PCSK9 inhibitors.
Introduction: products with consumer-dependent value are common, but drugs present special challenges
Even within a single indication, drugs provide different benefits to different people. To give a simple example, the value of a statin to a patient who has had a myocardial infarction and has a high cholesterol is demonstrably very large while the value of a statin to a patient with moderately elevated cholesterol and one other cardiac risk factor is real but much lower. This situation in which a product provides a different value to different consumers occurs frequently, and smart marketers have invested considerable effort designing methods to capture the most value from each customer segments. However, for a number of reasons, most of these approaches do not work for drugs:
- The common technique of deploying variants of the product to capture differential value of multiple consumer segments is not feasible;
- End-consumers are highly insulated from decision-making on product utilization – first through the providers, and then through payers;
- Finally, contracting with large public entities (e.g. Medicaid) often includes “lowest price clauses” which means that if a deep discount is offered to a customer segment, then it has implications for large swaths of the market.
As a result, what tends to occur is that pharmaceutical pricing is set under an “optimistic” value scenario. Given the very high cost for some therapies, the natural response from payers is to impose stringent limits on utilization with pre-authorization schemes to ensure that costs are controlled and only the patients who get the most benefit from the drug get it. The end result is that it is often the case that a large number of patients who could actually get moderate benefit are shut-out. Arguably this has occurred for PCSK9 inhibitors which priced at $14,000/year/patient have had a low level of uptake, and for the latest hepatitis C antivirals which have been limited to a rather small subset of patients that could benefit from them.
There have been some attempts by the biopharmaceutical industry to mitigate this through risk sharing value based arrangements, for instance, reimbursing treatment in case of failure. But these are relatively minor adjustments in the big picture of the health value left on the table through lack of access. Likewise, rebates and discounts agreements do not really address the core issue that by setting a high price, a drug manufacturer loses a portion of the eligible patient population, and that by setting a low price, a drug manufacturer fails to capture the value it provides to patients for whom the drug is highly beneficial. In what follows, we sketch out an alternative approach.
Drug pricing and population health
Across the population that falls within the approved treatment label of a drug, there is usually a spectrum of characteristics (demographic, disease progression, etc.) that modulates the benefit derived from using the drug. Typically, the exact magnitude of the variation of the benefit is not precisely known, especially early on in the clinical experience with the drug, but usually there is pretty broad consensus on the ordering, i.e. who benefits more, and who benefits less. Take for example a cholesterol-lowering medication; within the population included in the treatment label, some patients will be diabetic, some will be hypertensive, some will be smokers, etc. and among those there is a commonly accepted notion that those with higher cardiovascular risk will benefit most from cholesterol lowering treatment. The question is then: what is a rational way to set pricing in this context?
A classic approach: Fixed individual treatment pricing
Given a fixed individual treatment price (i.e. all treatments cost the same regardless of benefit), how would a company approach this from a value pricing perspective to maximize the return on the investment of discovering and developing a new drug? It would go something like this:
- Decide on an economic value of the health benefit: say $1.5M per major cardiovascular event avoided;
- Take an educated guess at the stratification of the population covered by the label according to cardiovascular risk and for each risk segment, estimate the probable benefit of treatment;
- Iteratively set the price to the health benefit for each segment and project the economics:
- The highest risk segment would correspond to the highest price and the lowest number of treatments (because the lower risk segments would not meet the value threshold at the highest price),
- The lowest risk segment would correspond to the lowest price and the highest number of treatments;
- Somewhere between these two extremes, there is a segment corresponding to a price that maximizes overall income; select this price.
From a payer perspective, the natural response will be to design pre-authorization rules that will screen out the segments below the value threshold and keep in those above.
This is depicted in Exhibit 1 where this type of analysis leads to pricing that keeps the four highest risk segments within the value threshold.
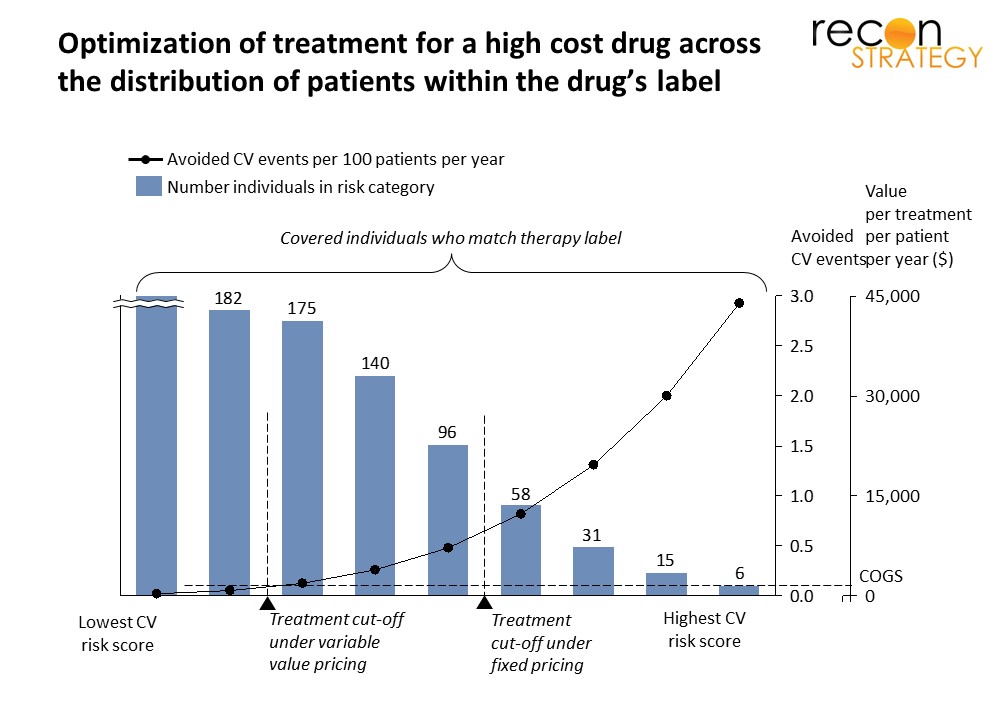
An alternate approach: Variable population-based pricing
An alternative is to vary pricing based on the value for each segment, but for a given covered population consolidated under a payer entity, derive an average price per treatment which will be directly linked to the aggregate value for the population to be treated. The pricing determination would go like this (with the two first steps similar as for fixed individual treatment pricing):
- Decide on an economic value of the health benefit: say $1.5M per major cardiovascular event avoided.
- Take an educated guess at the stratification of the population covered by the label according to cardiovascular risk and for each risk segment, estimate the probable benefit of treatment
- For each segment, set the (virtual) price to the health benefit
- Set the treatment cutoff to either
- The segment where the value of the health benefit is less than the COGS
or, - The segment where the health benefit is too small to be material
- The segment where the value of the health benefit is less than the COGS
- Take a volume-weighted average of the price over all the included segment to obtain the population-based pricing for that payer
Unlike the individual treatment pricing scheme, the variable population-based pricing will in most cases require explicit collaboration and negotiation between the payer and the drug manufacturer.
Exhibit 1 shows an example of the treatment cutoff with a high COGS (realistic for a biologic) covering many more risk segments than the individual treatment scheme pricing. This is of course because in this case, pricing to enable the lower risk patients does not cannibalize profit from the high risk patients for which the health benefit is very high.
Differences between the two approaches are summarized below for illustrative numbers:
*Because population pricing is the result of a negotiation between manufacturer and payer, we assume that the payer will claw back a part of the additional income going to the manufacturer by treating an expanded population. A plausible guess is that this will be a 50/50 split, and that is reflected in the adjusted values in the last three rows of the table.
Some options to operationalize population health drug pricing
There are many ways to do this but typically they will fall into one of two categories:
- Up-front agreement on a price-volume relationship based on the characteristics of the covered population
- An initial pricing estimate which is trued up at the end of the period using actual data on characteristics of the individuals who have used the drug
Up-front agreement
In this approach, the assumption is that payer and manufacturer can agree on an estimated distribution of risk and health benefit in the covered population. There can then be a spectrum of arrangements which essentially boil down to variants of a pre-agreed price volume relationship (with the assumption that at a given volume, the members with the highest risk and health benefit from the drug are treated first) so the average treatment price decreases monotonously with the number of patients treated. The advantage of this approach is that it is relatively simple – however it requires that the payer have a deep a priori understanding of the characteristics of the covered population and associated provider treatment behavior.
End of period true-up
This situation meets the three necessary conditions previously laid out[1] for payer/biopharma risk-sharing to work:
- Uncertainty: because there is uncertainty of who will use the drug
- Win-win: a value proposition that enables both the manufacturer and the payer to see some upside
- Arbiter of value: A viable approach to collect data, determine outcomes, and attribute value
For this approach, payer and manufacturer need to come to agreement on a formula that quantifies the health benefit of treatment and associated $ value based on patient characteristics. Given that, a guess is made on what the average treatment price will be as a place-holder to use during the period. At the end of the period, using clinical information collected through the pre-authorization process for treatments, the aggregate drug payments are trued up to match the actual patient population who received the drugs. I.e. if they were lower disease risk/health benefit than expected, there will be a refund from the manufacturer to the payer, and vice-versa if they were higher disease risk/health benefit than expected. This is a more complicated process than for the up-front agreement approach, but it does not require such an a priori accurate estimate of the characteristics of the population that will be treated going forward.
Final thoughts
An approach to drug pricing that recognizes that the magnitude of the health benefit for treatment varies within the population covered by the drug label has the potential to lower the average price per treatment, increase access and health impact, and improve return on investment for the manufacturer. It however requires a non-trivial effort to implement which makes it a plausible approach for only some drug treatments: those that are both expected to lead to large spend and for which there is significant heterogeneity of benefit in the population covered by the indication.
For this approach to work practically, one cannot be hung up on an absolutely accurate quantification of the health benefit per segment since this is clearly not achievable – the most one can hope for here in any circumstances is an estimate that will be better than the assumption of uniform value that underlies the traditional fixed price approach. That estimation needs to come from a collaboration between the holder of the clinical development data (the drug company) and the holder of the population data (the payer). But where the real bargaining comes in between the two is the overall factor converting these estimates to dollars.
[1] Biopharma risk-sharing: what needs to happen; https://reconstrategy.com/2016/04/biopharma-risk-sharing/